
Table 1: Quantitative comparison of the CAOS benchmark with related datasets. Thus, much future work remains to develop effective techniques for anomaly segmentation. The maximum softmax probability (MSP) scores only partially detect this anomaly, and there are many false positives.

The CAOS benchmark datasets and code for experiments is available at 2 Related Work Figure 1:Īn example image from the StreetHazards dataset with a sheep anomaly in view. As multi-label is a more natural setting than multi-class, this is an unexplored avenue that could grow in importance as research on out-of-distribution detection moves to large-scale, realistic evaluations. Finally, we explore the use of multi-label classifiers for out-of-distribution detection and evaluate several novel detection mechanisms in this setting. Hence we introduce a KL-divergence-based matching technique to improve standard out-of-distribution detection. We identify that the previous state-of-the-art out-of-distribution detector, when scaled up to datasets with similar classes, provides less meaningful anomaly scores. Large-scale datasets such as ImageNet-1K and Places365 In addition to introducing the CAOS benchmark, we also explore large-scale settings for more traditional whole-image anomaly detection. In large-scale out-of-distribution detection and segmentation. These novel baselinesĪlong with our anomaly segmentation benchmark open the door to further research Setting of multi-label out-of-distribution detection. Multi-class datasets and introduce detectors for the previously unexplored Variety of anomalous objects are naturally integrated, unlike before.Īdditionally, we improve out-of-distribution detectors on large-scale Simulated driving environment, we ensure the background context and a wide Ourīenchmark combines two novel datasets for anomaly segmentation that incorporateīoth realism and anomaly diversity. We propose to segment only the anomalous regions withinĪn image, and hence we introduce the Combined Anomalous Object Segmentationīenchmark for the more realistic task of large-scale anomaly segmentation.
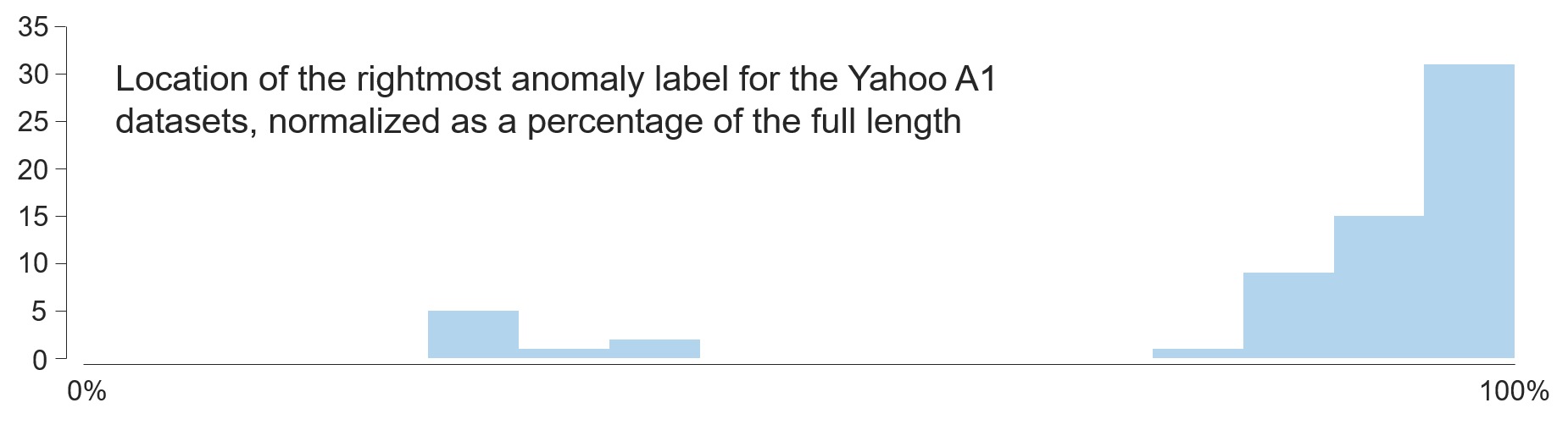
Research mainly focuses on small-scale images where the whole image isĬonsidered anomalous. Machine learning applications such as self-driving vehicles. Detecting out-of-distribution examples is important for safety-critical
